The automotive industry is undergoing a profound transformation, driven by advances in automation, digitalization, and sustainability. As one of the leading players in the sector, Gestamp continuously seeks innovative solutions to enhance production efficiency and quality.
This article explores Gestamp’s pilot use case, highlighting how the company leverages cutting-edge technologies to optimize manufacturing processes and stay competitive in a rapidly evolving market.
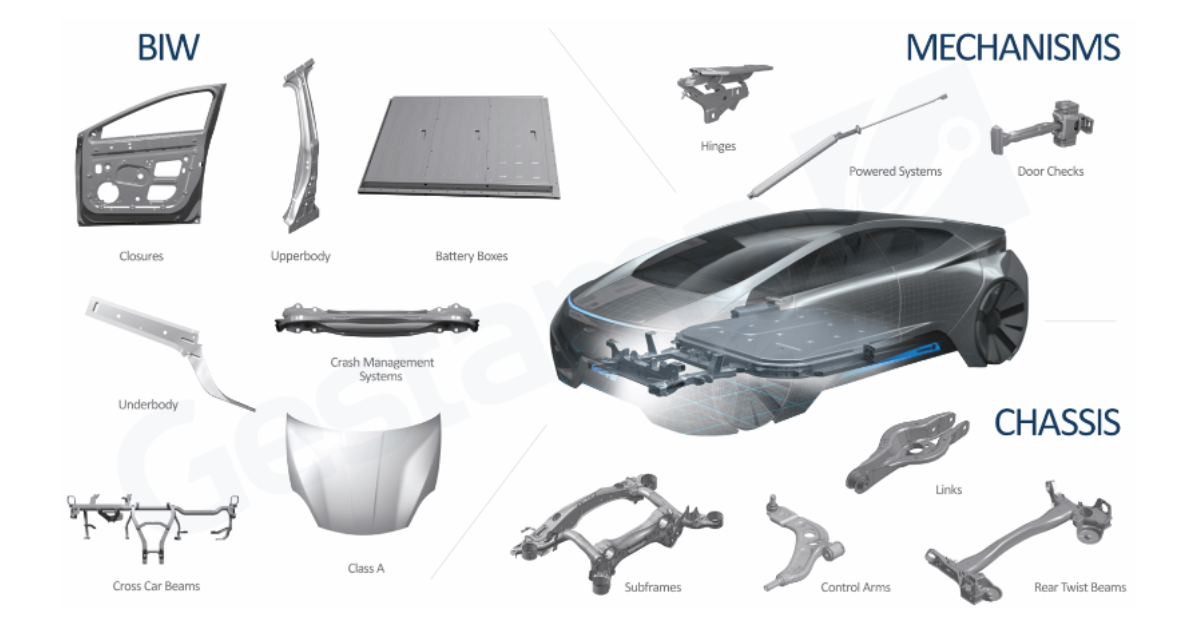
Gestamp specializes in the design, development, and manufacturing of metal components for leading automobile manufacturers. As vehicle designs become more complex and lightweight materials gain prominence, traditional manufacturing approaches must adapt. The company identified several key challenges, including:
- Enhancing precision in metal forming processes
- Reducing waste and improving material efficiency
- Increasing production flexibility to accommodate new vehicle models
- Streamlining quality control through advanced data analytics
Gestamp Pilot Use Case within the R3GROUP project
To address these challenges, Gestamp launched a pilot project integrating innovative technologies into its manufacturing workflow.
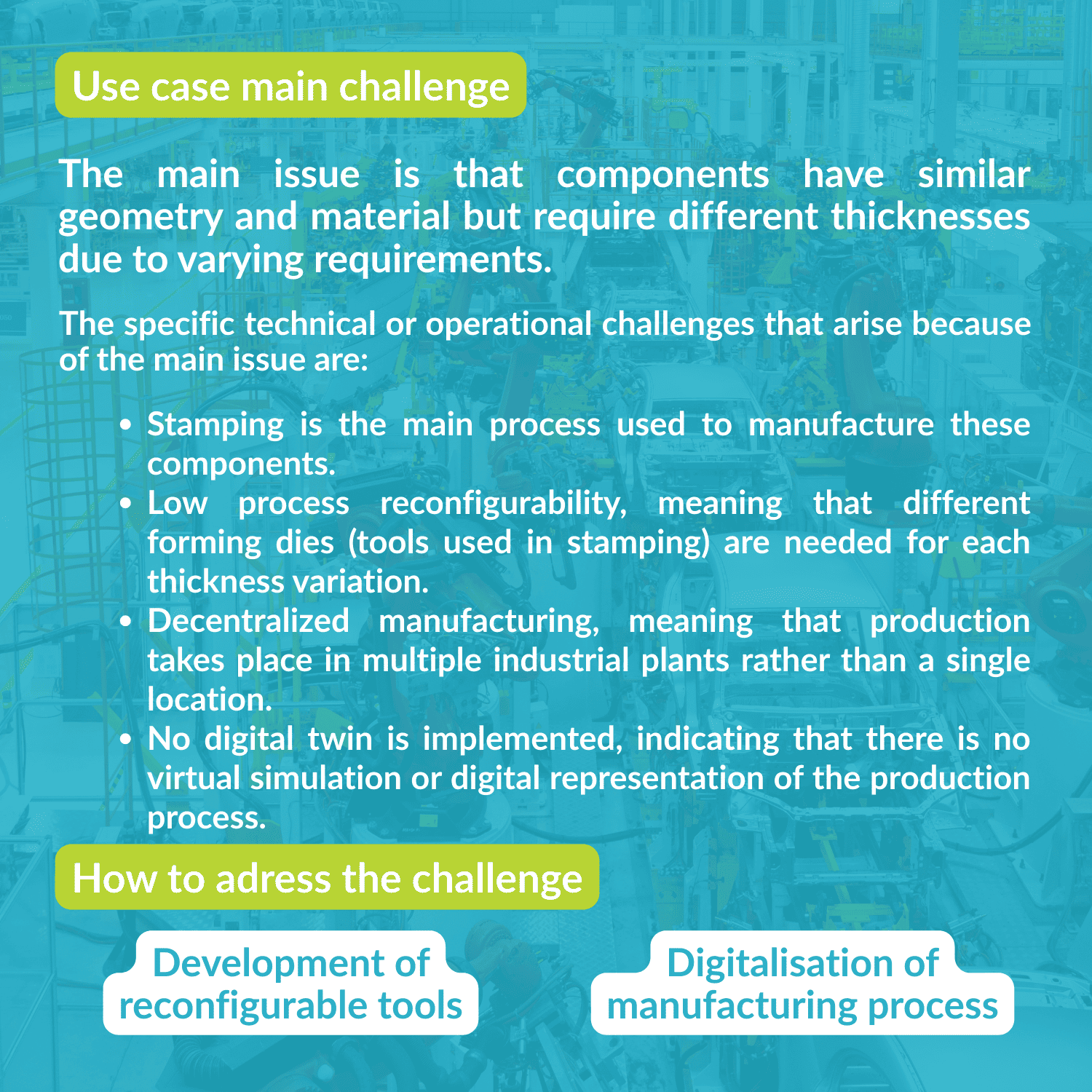
Gestamp is tackelling the challenge of producing control arms with the same geometry and material (CP800) but different thicknesses—3.4 mm and 3.7 mm. These variations require different forming tools and mechanical responses.
By combining simulation models and mechanical tests, Gestamp identified a critical region where thinner parts underperformed in buckling tests. To address this, they are reinforcing the 3.4 mm component, improving structural integrity without changing the overall design or tooling.
This approach demonstrates how targeted reinforcement and digital validation can increase manufacturing flexibility, reduce retooling, and support agile production in the automotive sector.
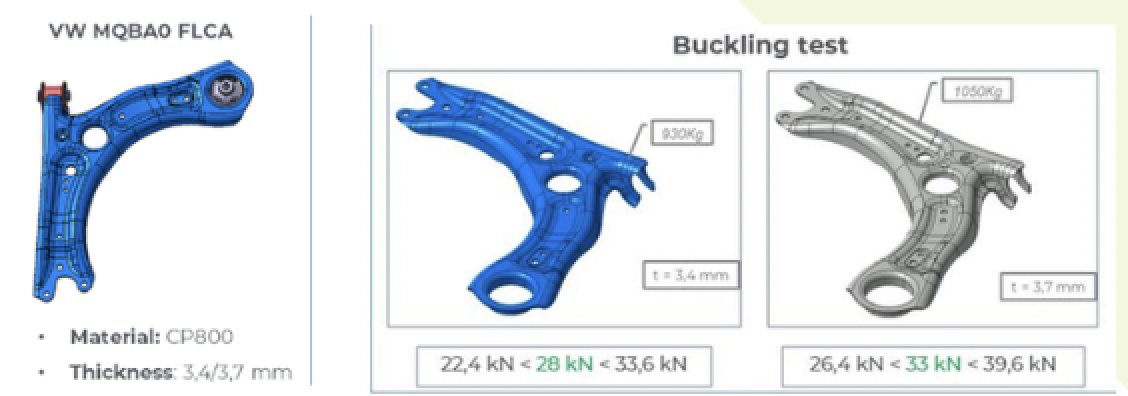
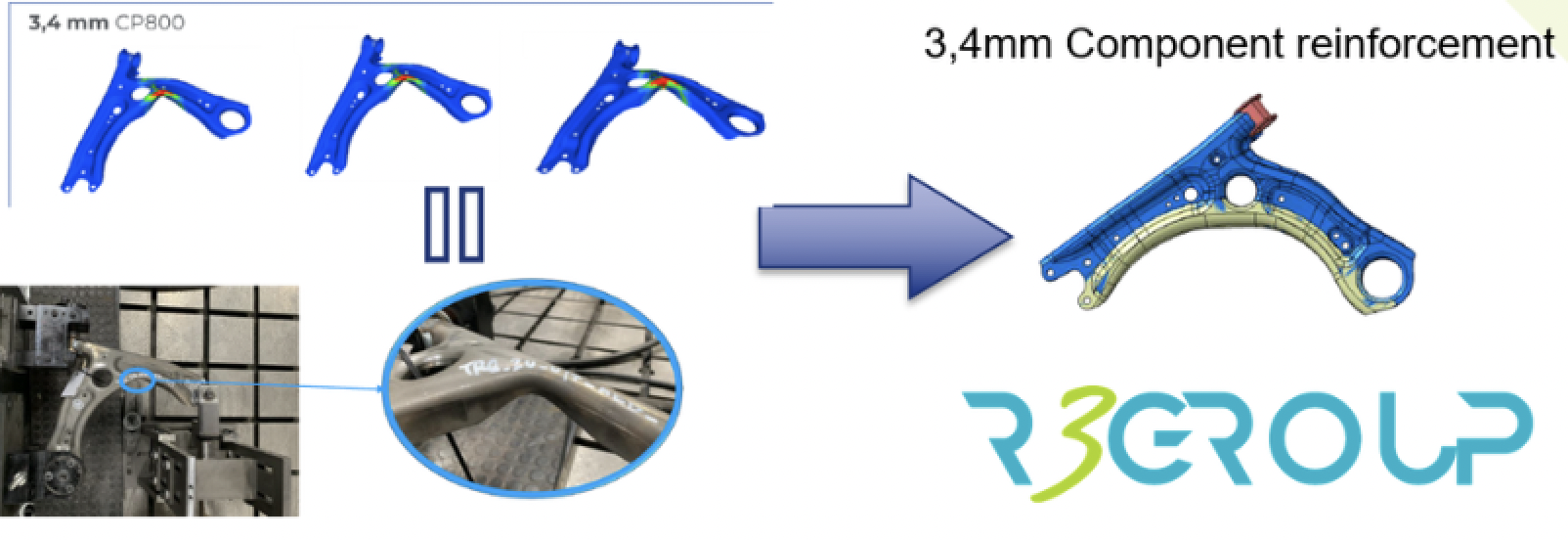
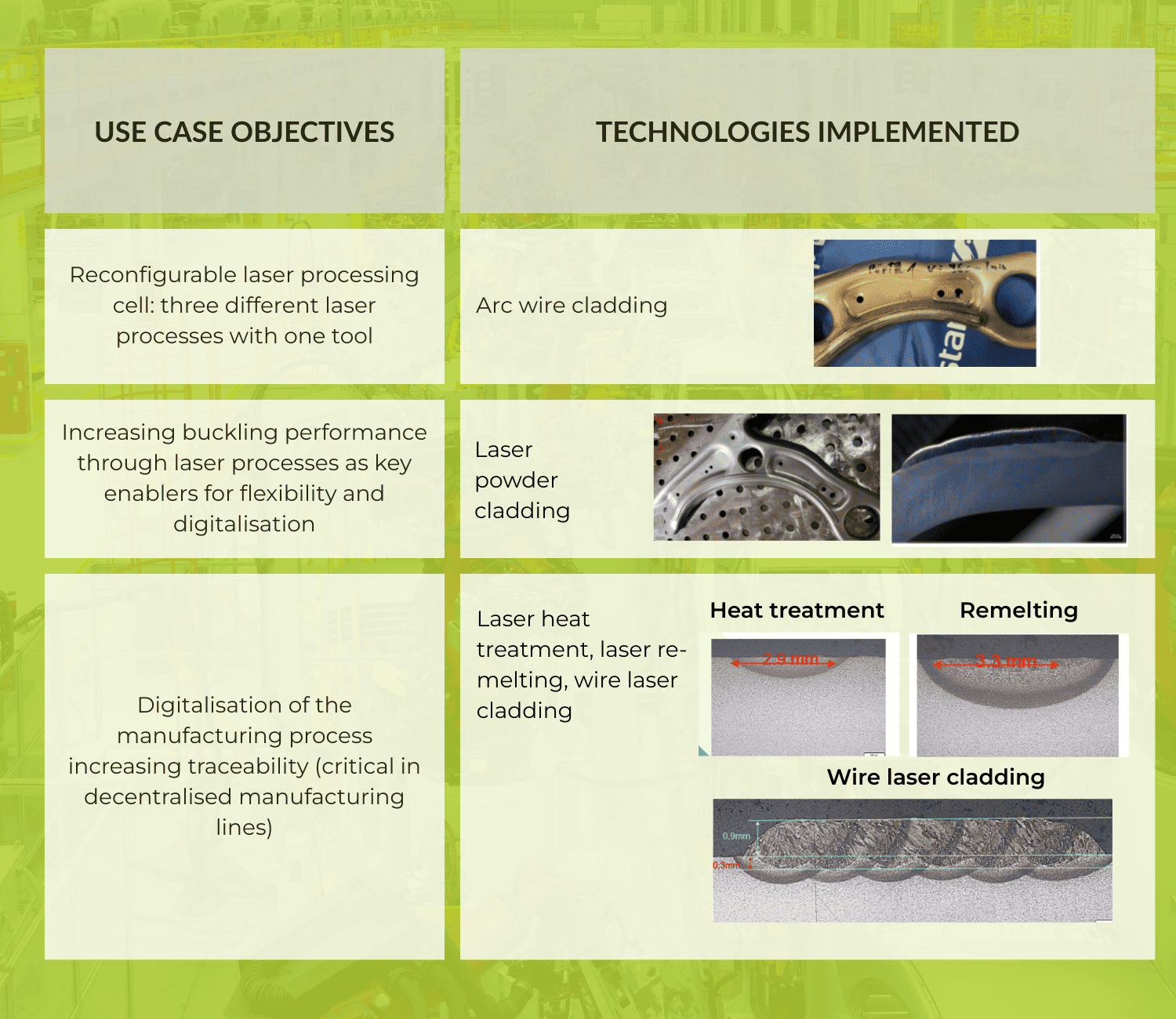
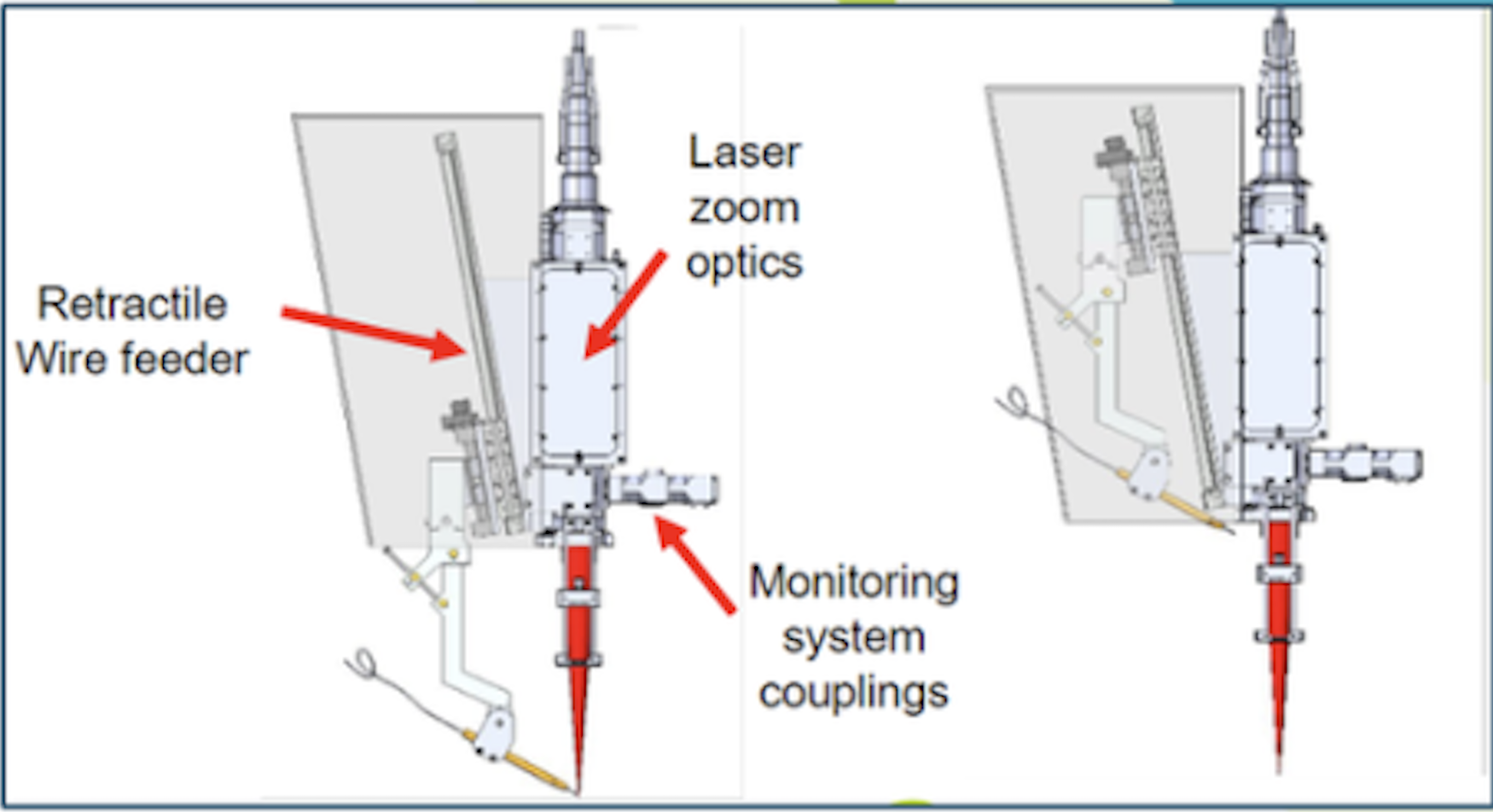
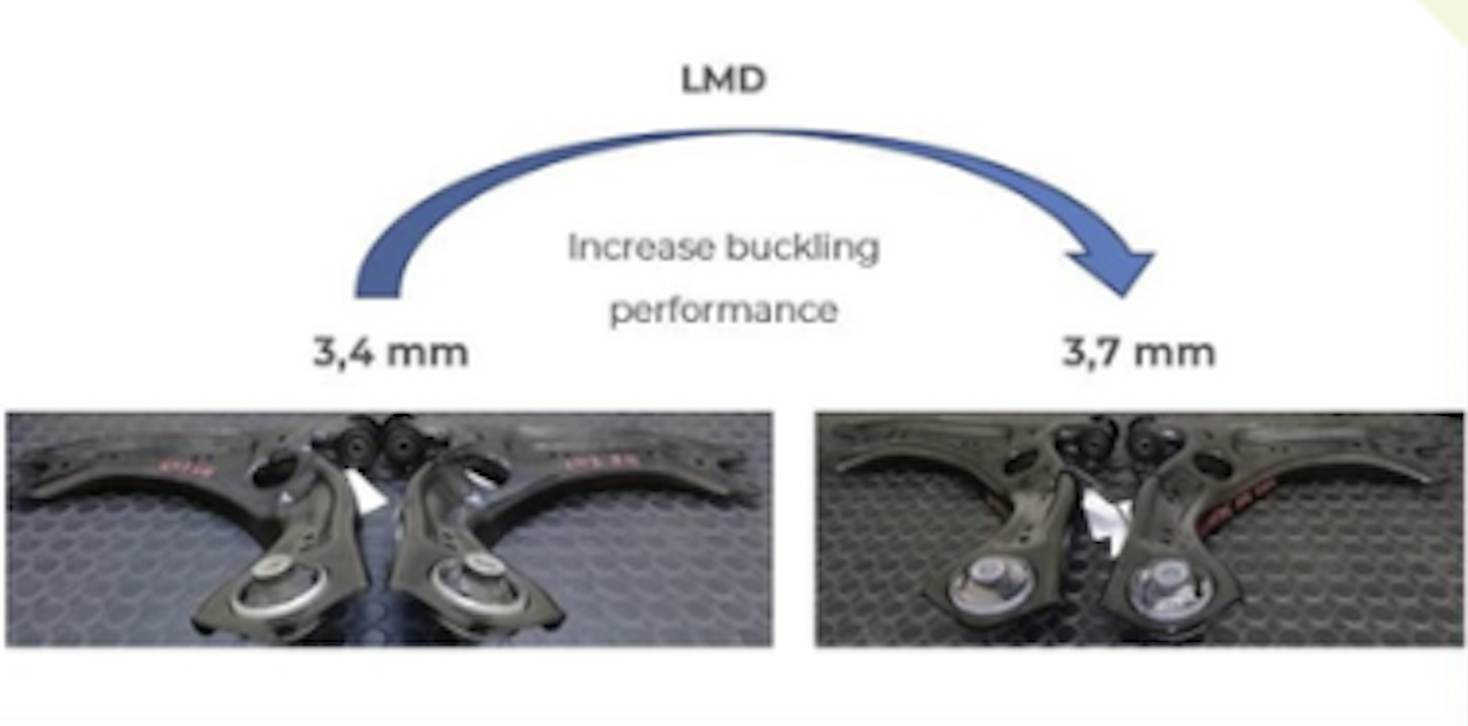
The core aspects of the initiative include:
Smart sensing and data-driven decision making
Gestamp implemented a network of smart sensors across its production lines to collect real-time data on material behavior, machine performance, and environmental conditions. This data-driven approach allows operators to make informed decisions, reducing defects and optimizing resource allocation.
Advanced robotics and automation
The company introduced adaptive robotic systems that enhance precision in metal forming and assembly. These robots adjust parameters dynamically, ensuring consistent quality while reducing downtime associated with manual interventions.
Digital twin technology
A digital twin—a virtual replica of the production environment—was developed to simulate and optimize processes before implementation on the shop floor. This technology enables predictive maintenance, minimizes trial-and-error iterations, and enhances overall process reliability.
Artificial intelligence in quality control
AI-driven image recognition systems were deployed to detect surface defects in manufactured components. By leveraging machine learning algorithms, Gestamp improved defect detection rates and reduced the need for costly rework.
Results and impact
The pilot implementation is yielding significant improvements across various manufacturing metrics:
- Higher production efficiency: Automation and AI-powered monitoring reduced production cycle times.
- Improved product quality: Advanced sensing and digital twin simulations enhanced manufacturing precision.
- Reduced material waste: Data-driven optimizations resulted in better resource utilization.
- Greater flexibility: The ability to quickly adapt production lines supports evolving automotive design requirements.
To become more specific, the integration of the modular, laser-based production equipment, along with the digital toolkit of the project (DTs, monitoring and control systems) and their link with the project platform are the key enablers for reconfigurability in the GESTAMP use case.
As a consequence, the following expected impacts are foreseen:
- Decrease by more than 50% the number of tools needed for the different family-related components (including welding, positioning and forming tools)
- Reduction of reconfiguration times related to tool changes of more than 50%.
- No influence of reconfiguration capabilities on the cycle time production of the components.
- Increase the availability of facilities by 5% by production shift.
- Improving flexibility by reducing the forming by up to 50%. Material saving: the weight of each forming die is around 5000kg. Reduction of maintenance and storage of dies.
Following the success of the pilot, Gestamp plans to scale these technologies across multiple production facilities. The company is also exploring further advancements, such as integrating blockchain for supply chain traceability and expanding AI applications in predictive maintenance.
Gestamp’s pilot use case exemplifies how embracing smart manufacturing technologies can drive efficiency, sustainability, and competitiveness in the automotive industry. As the sector continues to evolve, such innovations will be crucial in shaping the future of automotive manufacturing.
Do you want to know more about the R3GROUP technologies and how they become an early adopter programme?